October 20, 2019
Conference Proceedings, Hotel Grand Hyatt, Kochi, India
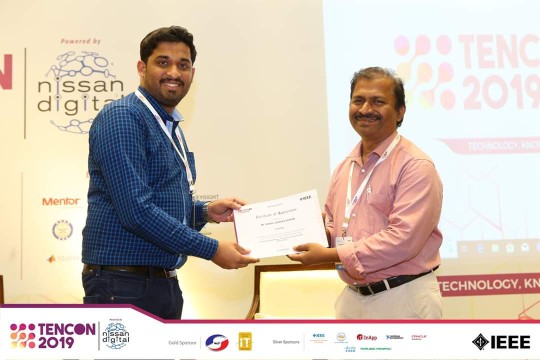
Conference proceedings on our paper: “Improving Histopathology Classification using Learnable Preprocessing”
Abstract:
A deep learning classifier trained on a source dataset often performs poorly on a target dataset, even for the same classification task, due to the differences in the distributions of the two datasets. In histopathology, the problem of dataset bias is even more severe due to the differences in specific tissue preparation and imaging set ups across patient cohorts. With the objective of improving the generalization across datasets, we propose a set of learnable preprocessing operations - an approach that has not been extensively explored - for a supervised deep learning framework that can be trained separately or together with the rest of the neural network. Through preprocessing, the data from a target domain is transformed before being fed to a classification module trained on the source domain to increase the overlap of the former's distribution with that of the latter. Through an extensive set of experiments on histopathology and face datasets, we show the particular and general utility of the proposed preprocessing operations for domain adaptation and compare it to previous approaches.